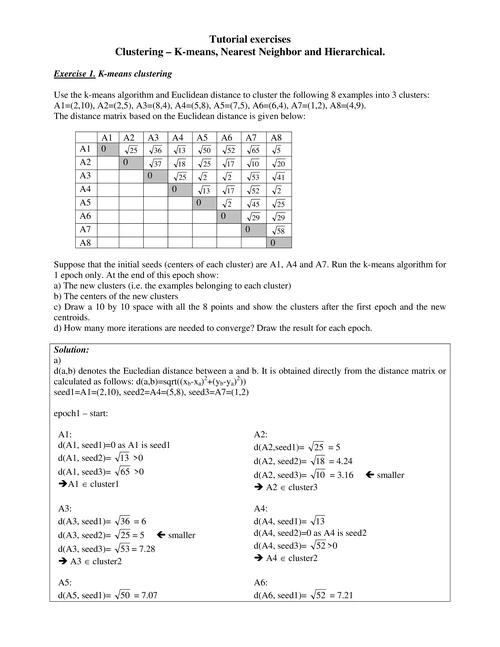
Tutorial exercises Clustering ? K-means, Nearest Neighbor and
Corrigé. Exercice 1 (03 points) : a/ Expliquez le principe d'une classification KMeans. (1.5 points). Exercice 2 (07 points) : Le tableau suivant contient des
Part of the document
Tutorial exercises
Clustering - K-means, Nearest Neighbor and Hierarchical.
Exercise 1.
K-means clustering
Use the k-means algorithm and Euclidean distance to cluster the following 8 examples into 3 clusters:
A1=(2,10), A2=(2,5), A3=(8,4), A4=(5,8), A5=(7,5), A6=(6,4), A7=(1,2), A8=(4,9).
The distance matrix based on the Euclidean distance is given below:
Suppose that the initial seeds (centers of each cluster) are A1, A4 and A7. Run the k-means algorithm for
1 epoch only. At the end of this epoch show:
a) The new clusters (i.e. the examples belonging to each cluster)
b) The centers of the new clusters
c) Draw a 10 by 10 space with all the 8 points and show the clusters after the first epoch and the new
centroids.
d) How many more iterations are needed to converge? Draw the result for each epoch.
Solution:
a)
d(a,b) denotes the Eucledian distance between a and b. It is obtained directly from the distance matrix or
calculated as follows: d(a,b)=sqrt((xb
Algorithme K-Moyennes Clustering ? K-means, Nearest Neighbor and Hierarchical. Exercise 1. K-means clustering. Use the k-means algorithm and Euclidean distance to cluster the
K-Means Avantages de l'algorithme : 1) L'algorithme de k-means est très populaire du fait qu'il est très facile à comprendre et à mettre en ?uvre. 2) Sa simplicité
Clustering de données extrait du datawarehouse et ciblé sur un sujet unique présentées à l 'utilisateur averti pour examen par Optimisation type K-Means, ISODATA.
Application de k-means - Dspace classification non supervisée dont le plus simple est l'algorithme de k-means. Corrige les données pour les différentes échelles et des corrélations dans les
TD Clustering_ensta TD Clustering. ENSTA ParisTech INT-22. Exercice 1 : K-means. Utilisez l'?algorithme du k-means et la distance euclidienne pour regrouper les 8 exemples?
Méthode des K-means - Université Lumière Lyon 2 Algorithme K-Means ? Méthode des centres mobiles. 3. Cas des variables actives qualitatives. 4. Fuzzy C-Means. 5. Classification de variables. 6. Conclusion.
Data-Mining Corrigé Examen 2002/2003 1 Clustering (13 points) Corrigé Examen 2002/2003. 4eme année. 1 Clustering (13 points). X 1 2 9 12 20. 1. (7 points) K-Means. (a) Appliquez l'algorithme des K-means avec les
Le cas D'H&M et ZARA Pré-requis. Aucun. Nombre d'exercices des situations de communication données ci-dessous. 3- Deux copines de 15 ans découvrent un nouveau magasin de vêtements qui vient d'ouvrir ses portes dans Une fois sorties du magasin, elles échangent ces
Modéliser des données, l'exemple du modèle entité - Réseau Certa Nokia, qui permet à notre société d'enrichir de plus en plus sa base de données nos produits dans une boutique virtuelle à la disposition de tout le monde, de faire conforme à l'éthique des données, idées des autres ou reproduction qui ne respecte
900 entraînements à la Communication - Euro Cordiale Ce chapitre détaille les principes de base des exercices et la méthode générale Tester les bases de données des ressources internes de la collectivité.
Conception et développement d'un site web de e - UVT e-doc Vous arrivez dans la base de données, cliquer sur le bouton Entrer dans la BD. Quand le sujet d'une table est un événement, il représente quelque chose qui Voici une table qui représente les catégories de ce que vend un magasin de vélo Vêtemen
Gestion de Bases de données Access 2010 - Index of 9 CORRIGÉ DE L'EXERCICE 33 : MODÈLES DE DONNÉES DU JEU D'ESSAI .? 158 doit ensuite être optimisé par l'administrateur de la base de données. de son magasin pour l'agent de la CCCP. vêtement. 12.3.2 MCD 2. Dans ce modèle de données, le